Deep learning algorithms are being increasingly used in contact centers as the adoption of these algorithms help them in improving the first-call resolution process, reducing call durations, curtailing total call volume, and enhancing customer satisfaction, thereby, increasing their business revenue. Deep learning algorithms assist in reducing the time required for problem resolution in contact centers by efficiently routing the calls to the concerned individual processing adequate knowledge.
Thus, the surging number of contact centers, owing to the expansion of end-use industries, will fuel the adoption of deep learning algorithms in the coming years. Moreover, the rising need for drug discovery will also drive the deep learning market at a CAGR of 35.2% during the forecast period (2020–2030). The market was valued at $3.7 billion in 2019 and it is projected to generate $102.4 billion revenue by 2030.
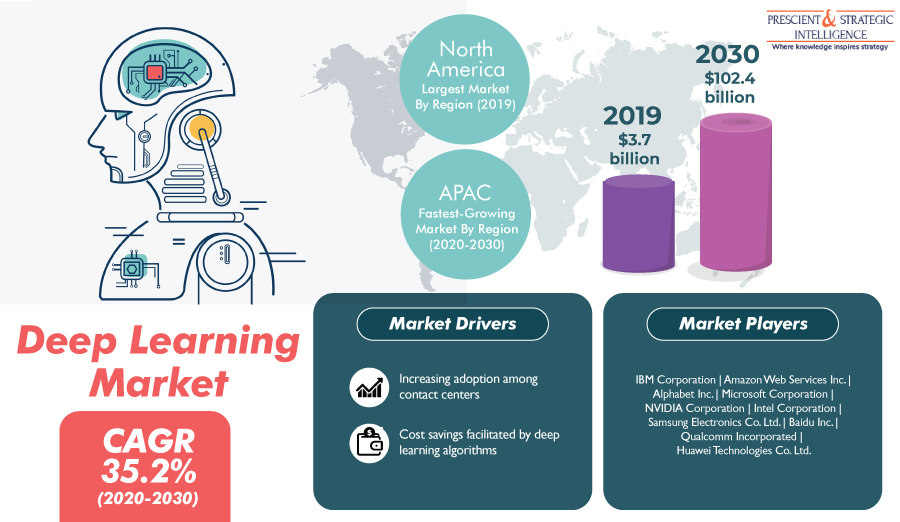
Get the Sample Copy of this Report @ https://www.psmarketresearch.com/market-analysis/deep-learning-market-report/report-sample
The healthcare industry uses deep learning solutions for drug discovery and development by understanding the medicinal properties of novel compounds and allowing deeper analysis of new materials. Apart from this, deep learning solutions also help medical professionals recommend the best treatment for patients by analyzing their medical history in detail.
The increasing adoption of deep learning software in the healthcare industry and contact centers helps in reducing operational costs. Deep learning is concerned with the exploration and creation of algorithms that allow computers to constantly learn and adapt. The complicated relationship between data sets usually makes it difficult to implement analysis methods such as hypothesis testing and regression analysis.
In these situations, the deep learning software allows classification and systematic coding of data sets, which further enhances visibility in terms of errors in datasets and reduces the need for human intervention in analysis.